1. Complex systems and infrastructures, cyber physical systems
The analysis of these systems cannot be carried out only with classical methods of system decomposition and logic analysis. A framework is needed to integrate a number of methods capable of viewing the problem from different perspectives (topological and functional, static and dynamic, discrete and continuous...), properly treating uncertainties by probabilistic and non-probabilistic methods. Our main contribution is to use stochastic processes, data driven approaches and Monte Carlo simulation to identify influent parameters and critical items, and to define proper level of abstractions for modelling. The modelling work is achieved in the perspective of providing decision indicators for Safety, Risk, Availability and Maintenance management with a careful quantification of uncertainties.
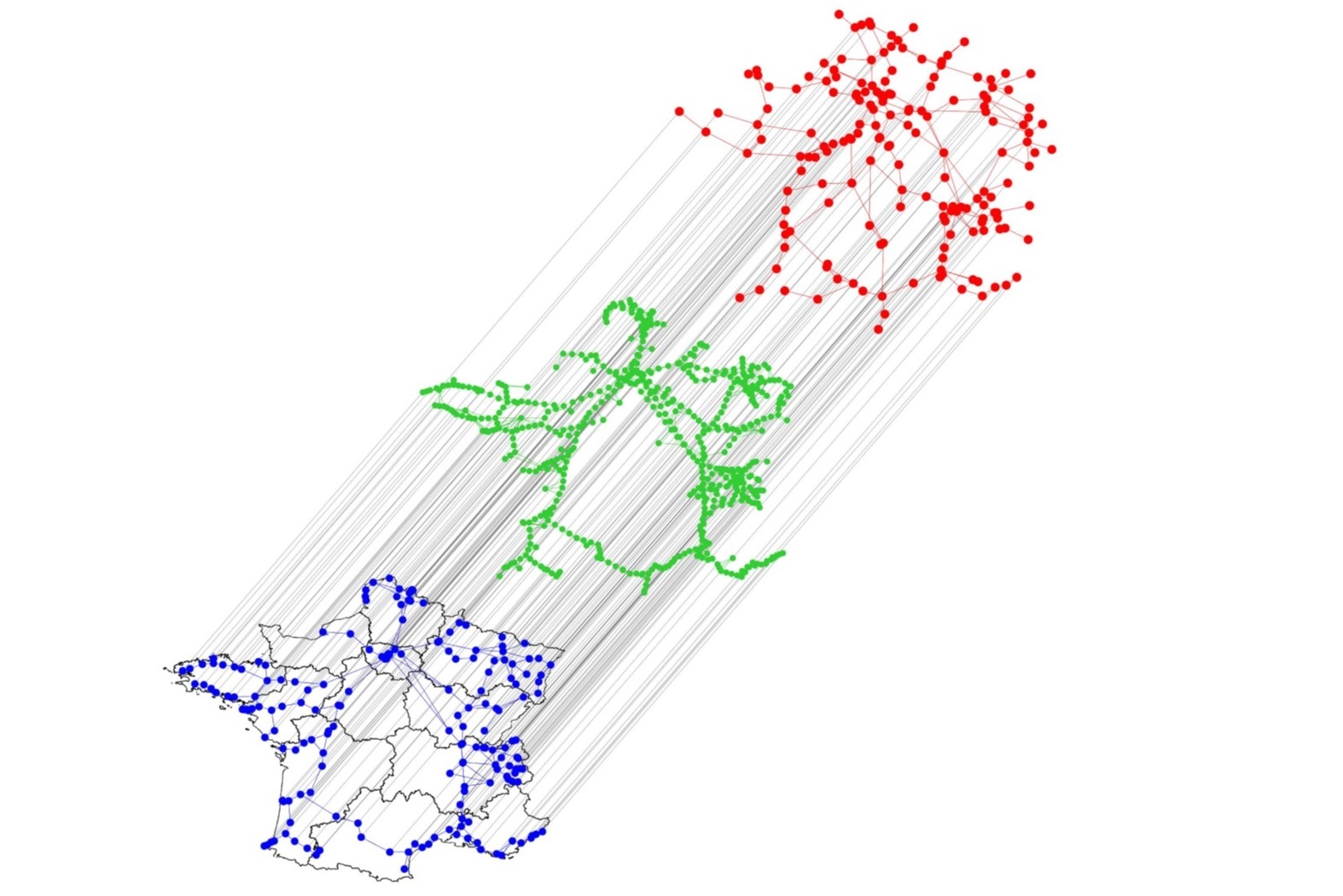
Related publications
Bellè, A., Zeng, Z., Duval, C., Sango, M., & Barros, A. (2022). Modeling and vulnerability analysis of interdependent railway and power networks: Application to British test systems. Reliability Engineering & System Safety, 217, 108091.
2. Industry 4.0 and predictive maintenance
In the framework of Industry 4.0, our main contribution is to develop advanced models and optimization methods for dynamic risk management and predictive maintenance. This encompasses the assessment and modelling of components degradation with system usage, and the analyze and optimization of maintenance solutions. This can be done by multi-state physics, Bayesian and Markov chains models, Monte Carlo simulation. A particular focus is on failure prediction and prognostics of critical components, by data-driven approaches, e.g. adaptive artificial neural networks, support vector machines and alike. Regarding maintenance optimization, dynamic decision-making techniques such as the Markov Decision Processes, Partially Observable Markov Decision Processes, and multi-stage robust optimization, are the focuses.
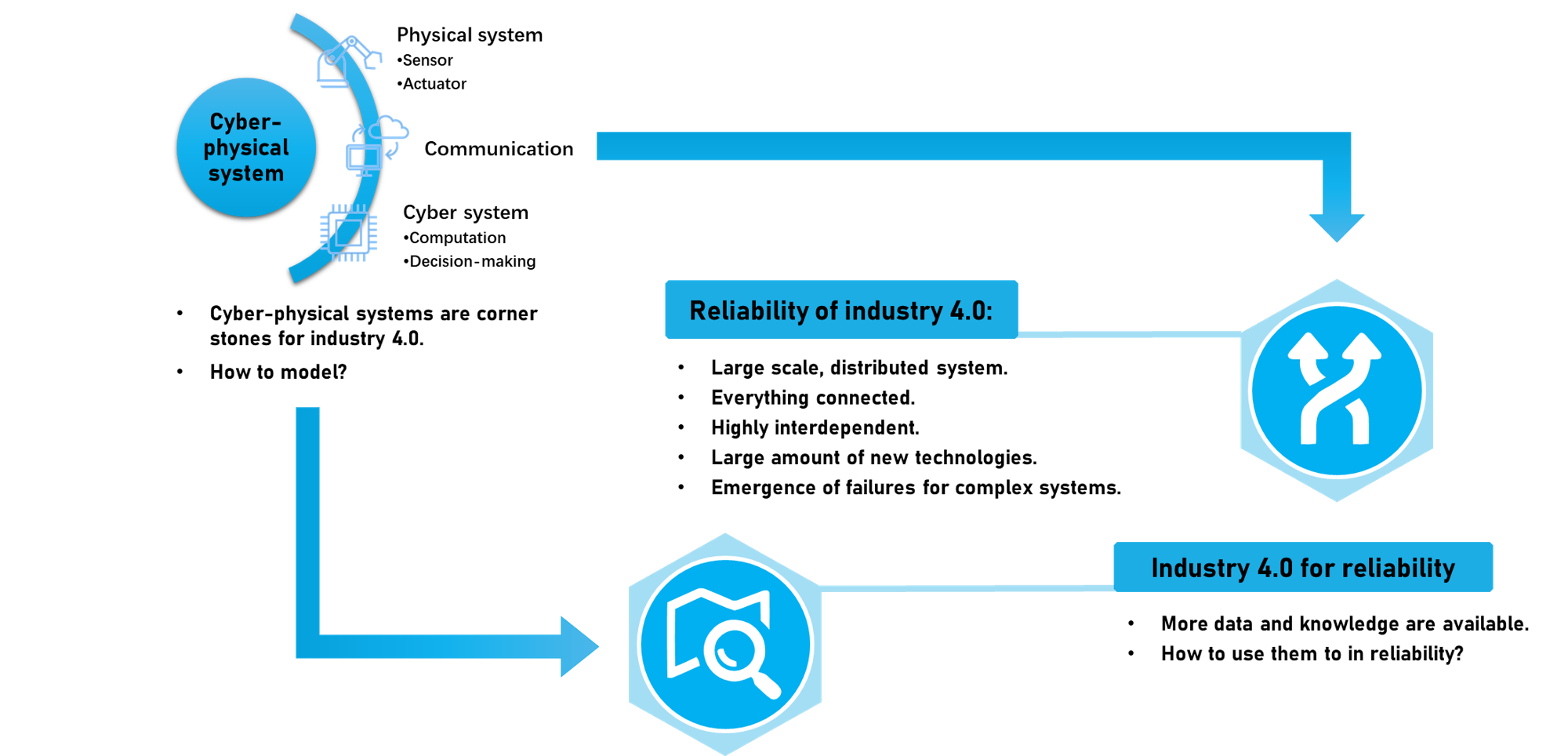
Related publications
Roux, M., Fang, Y. P., & Barros, A. (2022). Maintenance planning under imperfect monitoring: an efficient POMDP model using interpolated value function. IFAC-PapersOnLine, 55(16), 128-135.
Xiahou, T., Liu, Y., Zeng, Z., & Wu, M. (2022). Remaining useful life prediction with imprecise observations: An interval particle filtering approach. IISE Transactions, 1-16.
3. Resilience assessment and optimization
We intend to assess and optimize the resilience of complex systems and (interdependent) critical infrastructures by modeling and optimizing the processes of barrier management, mitigation, crisis management, recovery. One of the objectives is to guaranty business continuity by investigating in which degraded states the system should be put back in a minimal amount of time. The approaches developed are related to agent-based modelling, advanced simulation techniques, stochastic and robust optimization, and reinforcement learning.
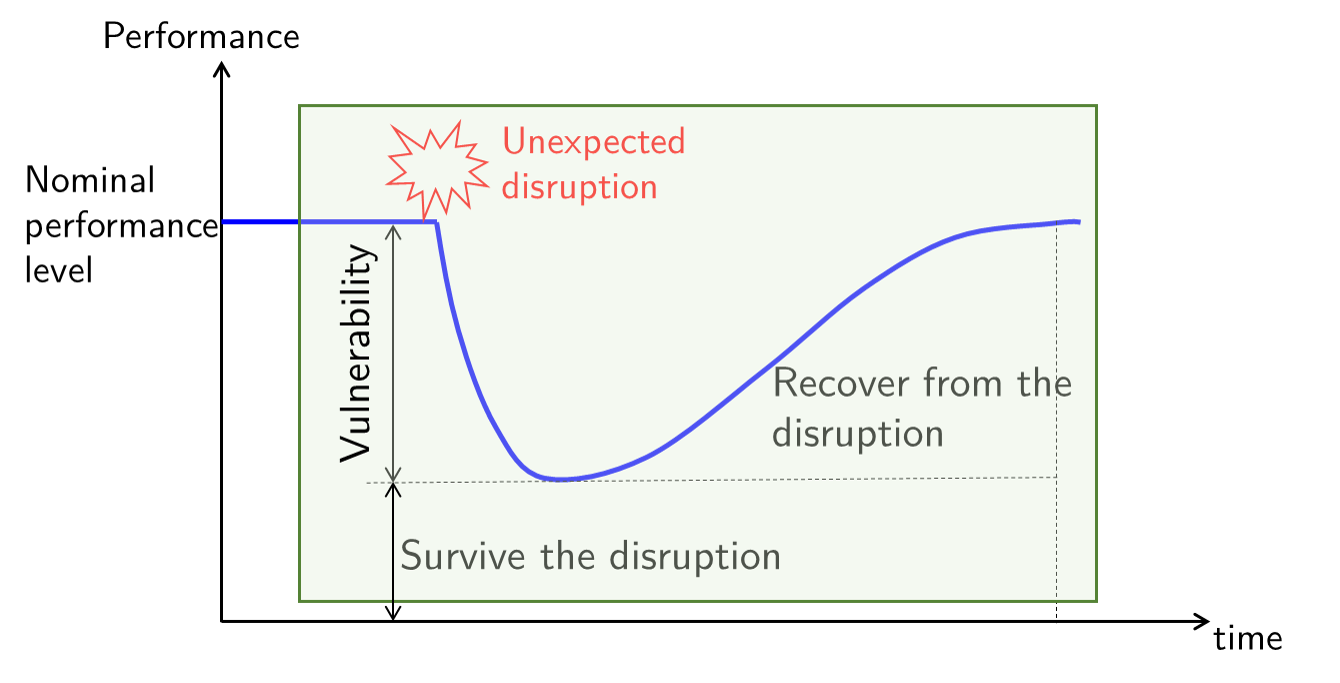
Related publications
Bellè, A., Abdin, A. F., Fang, Y. P., Zeng, Z., & Barros, A. (2023). A data-driven distributionally robust approach for the optimal coupling of interdependent critical infrastructures under random failures. European Journal of Operational Research.
Wang, H., Fang, Y. P., & Zio, E. (2022). Resilience-oriented optimal post-disruption reconfiguration for coupled traffic-power systems. Reliability Engineering & System Safety, 222, 108408.